Benchmarking Machine Learning Workloads On Emerging Hardware
The emerging MLPerf benchmark suite touts Time to Accuracy TTA as the metric. AIs rapid evolution is producing an explosion in new types of hardware accelerators for machine learning and deep learning.
Why Machine Learning Needs Benchmarks Sigarch
Benchmarking Machine Learning Workloads on Emerging Hardware.
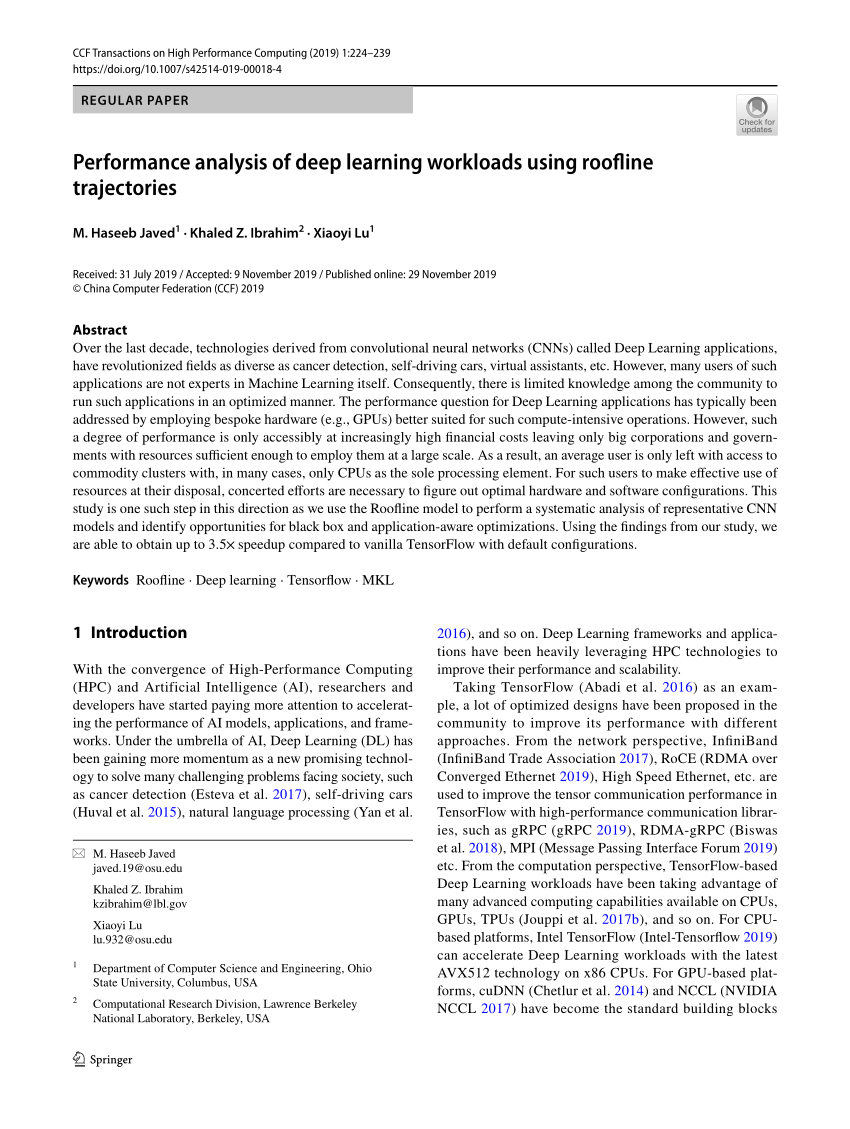
Benchmarking machine learning workloads on emerging hardware. Benchmarking Deep Learning Workloads on Large-scale HPC Systems AmmarAhmad Awan and Dhabaleswar K. With evolving system architectures hardware and software stacks diverse machine learning ML workloads and data it is important to understand how these components interact with each other. Tom St John Murali Emani.
With evolving system architectures hardware and software stacks diverse machine learning ML workloads and data it is important to understand how these components interact with each other. The major effort is driven from DoE labs namely LBNLNERSC Argonne and Oak Ridge. Well-defined benchmarking procedures help evaluate and reason the performance gains with ML workload-to-system mappings.
March 5 2021 With evolving system architectures hardware and software stacks diverse machine learning ML workloads and data it is important to understand how these components interact with each other. Deep learning DL is a popular branch of ML that poses unique challenges to ML systems due to its high demand and complex workload in. Benchmarking would help evaluate and reason the performance gains with workload.
This group is closely associated with the MLPerf organization. This comment is monitored to verify that the site is working. CMC Microsystems is pleased to organize the 2 nd workshop on accelerating AI highlighting the challenges and opportunities of AI acceleration from the cloud to the edge.
Benchmarking Machine Learning Workloads on Emerging Hardware. As the AI arena shifts toward workload-optimized architectures theres a growing need for standard benchmarking tools to help machine learning developers and. 0230 PM Orals.
AbstractBenchmarking for machine learning workloads should consider accuracy in addition to execution time or throughput. Secure and Resilient Autonomy. In this special guest feature Murali Emani from Argonne writes that a team of scientists from DoE labs have formed a working group called MLPerf-HPC to focus on benchmarking machine learning workloads for high performance computing.
With evolving system architectures hardware and software stack and scientific workloads and data from simulations it is important to understand how these interact with each other. With evolving system architectures hardware and software stacks diverse machine learning ML workloads and data it is important to understand how these components interact with each other. Well-defined benchmarking procedures help evaluate and reason the performance gains with ML workload-to-system mappings.
With evolving system architectures hardware and software stack scientific workloads and data from simulations it is important to understand how these interact with each other. Software-Hardware Codesign for Machine Learning Workloads. The Emerging Role of Cryptography in Trustworthy AI.
Well-defined benchmarking procedures help evaluate and reason the performance gains with ML workload-to-system mappings. Behnaz Arzani Bita Darvish Rouhani. In this paper we explore the advantages and disadvantages of different metrics that consider time and accuracy from the perspective of comparing the.
With the rapid growth of hardware and software HWSW innovation in machine learning ML there is a need for representative benchmarks to enable fair and reproducible performance benchmarks that can accelerate the development of new algorithms and ML systems. 2 a novel accelerator design paradigm with high-dimensional design space support and fine-grained adjustability to overcome the existing design drawbacks. Wed Mar 04 0700 AM -- 0330 PM PST Level 3 Room 6.
This need has emerged since machine learning is moving towards a workload-optimized structure. Benchmarking would help evaluate and reason the performance gains with workload to system mapping. Automated Machine Learning For Networks and Distributed Systems.
Chaired by Intel Corp EEMBCs Machine Learning Benchmark Suite group will use real-world ML workloads from virtual assistants smartphones IoT devices smart speakers IoT gateways and other. And 3 a design space exploration DSE engine to generate optimized accelerators by considering targeted AI workloads and available hardware. Benchmarking Machine Learning Ecosystem on HPC systems.
InProceedings of the Machine Learning on HPC EnvironmentsMLHPC17 in conjunction with SC 17 Denver CO. Key features include 1 direct support to popular machine learning frameworks for DNN workload analysis and accurate analytical models for fast accelerator benchmarking. This workshop aims to bring together experts from industry and academia to share their latest achievements and innovations in the field of AI and machine learning algorithms software and hardware from the.
As a result there is a more than ever need for standard benchmarking tools that will help machine learning developers access and analyze the. Benchmarking will help to better understand the model-system interactions and help co-design future HPC systems for ML workloads. The harbinger of the current AI Spring deep learning is a machine learning method using artificial neural networks moving vast amounts of data through many layers of hardware.
Software-Hardware Codesign for Machine Learning Workloads. Benchmarking Machine Learning Workloads on Emerging Hardware Co-located with MLSys 2020 Austin TX USA March 4 2020. CPUs dense many-cores are emerging CPUs exist on nodes with GPUs Many-core Xeon POWER9 EPYC ARM etc.
Performance Analysis Of Deep Learning Workloads On A Composable System Deepai
Digging Into Mlperf Benchmark Suite To Inform Ai Infrastructure Decisions
Huawei Atlas Ai Computing Platform A Gift For Developers Cio Huawei Traffic Analysis Development
Hpe Launches Apollo 6500 Gen10 System As Part Of Ai Solution Push
Digging Into Mlperf Benchmark Suite To Inform Ai Infrastructure Decisions
Ai Infrastructure Market Size Industry Analysis And Market Forecast To 2025 Marketsandmarkets
Http Austinclyde Com Challenge20 Paper 9 Pdf
Demystifying Mlperf Inference The Mlperf Community Is Enabling Fair By Anton Lokhmotov Towards Data Science
Intel Xeon Scalable Processors Artificial Intelligence
Digging Into Mlperf Benchmark Suite To Inform Ai Infrastructure Decisions
How To Measure The Performance Of Your Ai Machine Learning Platform
Artificial Intelligence Chipsets Market By Technology Hardware Covid 19 Impact Analysis Marketsandmarkets
Pdf Performance Analysis Of Deep Learning Workloads Using Roofline Trajectories
Machine Learning Workloads On Premises Vs The Cloud
Mlperf Hpc Working Group Seeks Participation Insidehpc
Https Www Vmware Com Content Dam Digitalmarketing Vmware En Pdf Solutions Vmware Ai Ml Ra Ma Pdf
Dlobd An Emerging Paradigm Of Deep Learning Over Big Data Stacks Wi
Post a Comment for "Benchmarking Machine Learning Workloads On Emerging Hardware"